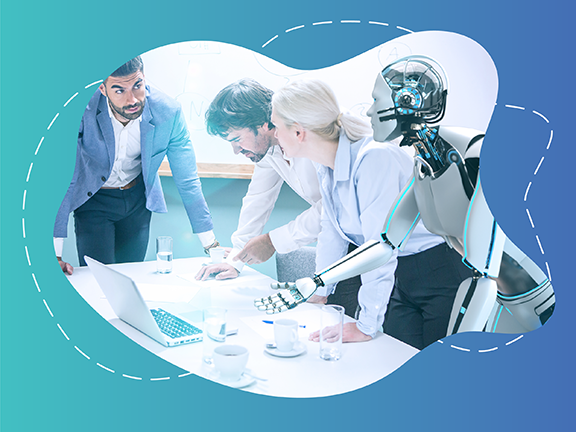
AI, UI, and You and I
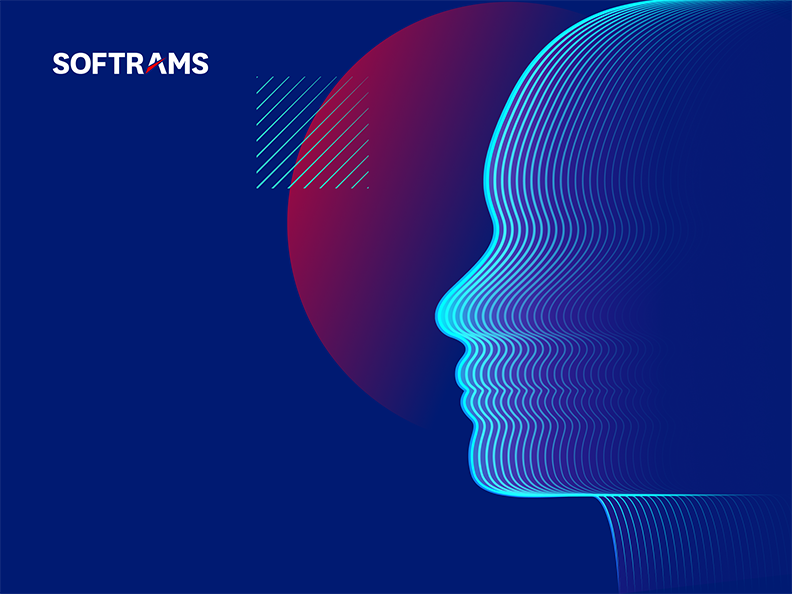
Softrams is deeply entrenched in work at CMS with a variety of prime contract programs. These CMS programs support significant numbers of users both external and internal to CMS. The web-enabled interfaces of these applications are extensive and some of our most significant achievements have been in the design and redesign of workflows in extensive collaboration with users and stakeholders. In these efforts to design human centered software we focus on the Human Experience – the needs, context, behaviors, and emotions of the people that the solutions will serve. Human Centered Design (HCD) is a primary focus for Softrams, as is innovation with artificial intelligence (AI) and machine learning (ML).
AI Opportunities and Impact
Organizational workforces continue to spend considerable time on administrative and internal facing activities – even after the implementation of IT systems that fulfill many data processing and related tasks. Some of this relates to the need for human intervention with unstructured data – information that AI approaches can now tackle. In all, AI is enabling organizations like CMS to refocus people resources even further on higher level strategic work and external facing consultative work with constituents and customers.
AI brings new capabilities to doing new things and to doing things differently. And, of course, this is not without impact. As AI is implemented, this will change business processes and affect the workforce, users, contractors, stakeholders, and the public as many other IT implementations have done so before. And in the context of these new business processes, humans are now interacting with a different type of system than before. So, it’s useful to explore the implications for human centered design work.
AI may be applied with varying degrees of AI support. One approach is decision support – where AI provides information and recommendations that support human performance. This is augmentation. AI can alternatively selectively filter what requires manual intervention – bypassing or completing some tasks but prioritizing other tasks for human action and decision. Finally, of course, some tasks may be nearly fully automated.
AI Helping Humans & Humans Helping AI
It’s true, that over the next decade, more work will be assigned to machines. However, in nearly all assessments, human-machine collaboration will still be required to effectively use AI. That is, AI augments humans. The fact is that there isn’t expected yet to be full AI replacement of human roles in business processes even with structured repetitive activities like filing documents. And the AI-human collaboration will be more significant for quantitative reasoning skills such as interpreting language, performing analytics, and software programming. And of course, cross-functional reasoning skills such as developing strategy and managing people are expected to be AI assisted in only limited ways.
The human-machine collaboration is bi-directional in that there is often a process of co-learning and co-adaptation. When people interact with AI systems, they can influence what the system will produce in the future. These systems that evolve over time alongside their users are often the most helpful. But as they change too, this also affects how the users interact with them. Human user roles lie in training the AI further, monitoring the AI’s performance, and helping to put the AI’s recommendations into a business context. In other words, I may have an AI at my disposal, but my AI has a human too. How do we best support users in these roles? For both sides of the coin – we have much work to do gain further experience on how these processes will operate, the roles and responsibilities, and the best designs for supporting both users and machines in these roles. And this is what human centered design research can address for us.
Human Centered Machine Learning
Let’s look at some of the areas in which Human Centered Machine Learning (HCML) must focus:
- Understanding Bi-directional Adaption: This has implications for human centered design. There is possibly a need to go beyond personas and examine the needs and interactions of both human and non-human actors. Some have suggested adopting Actor-Network Theory approaches for example.
- Designing for Trust: We rely on much to judge our trust of human experts – but not all of that is reliable. What information do we use and biases do we have in basing trust in an AI?
- Communicating AI Limitations: A top design challenge is communicating and teaching users that machines are limited and may be wrong. What interface designs help users to recognize the limitations of the AI? For example, displaying the level of confidence the AI has in its recommendation. It’s very important to weigh the costs of both false positives and false negatives and communicate them.
- Addressing Explainable AI (XAI): Deep learning models are largely a black box and questions of why recommendations are offered by the AI cannot be readily made apparent. Some common methods like Local Interpretable Model-Agnostic Explanations (LIME) leverage explainable machine learning models to simulate why an AI provided a particular answer. This makes it possible to design results displays as we have done to date for logistic regression models and other analytics.
- Working Around the Unknown: Prototyping AI systems can be difficult with the considerable training investments to be made to develop the solution. Some reports suggest that “Wizard of OZ” methods are seeing a resurgence after many years. By this approach, humans act behind the scenes to simulate the AI in the prototyping exercises.
Designing With Subject Matter Expertise
It’s clear that significant technical expertise is required for managing AI development. But it ia equally apparent, as with other analytics, that this development needs to be supported by understanding of the business domain areas and the users. This is the age-old problem in developing IT. It’s the product management balance of building the right product for business value and building the product right for usability.
Machine learning and AI applications still most often fail because user design is not done well. And business value requires expertise to achieve — model building holds risk in the lack of control of all factors. As an example, consider the data scientist who detects suppliers billing anomalous amounts of medical oxygen. However, the cases identified are exceptions. They are due to where the patients are living – in the mountains with high altitudes and thin air. Not necessarily an obvious finding – but unlikely to be revealed with the data scientist working alone. As we transform static business intelligence displays to interactive AI systems, the key ingredient will be training the AI to recognize business significance – when trends and events are meaningful.
Final Thoughts: Managing AI Change
Ultimately our key decisions are human ones that an AI cannot necessarily answer:
- What are the business problems we need to solve? We need to use real intelligence here, not the artificial stuff.
- How can I get a solution to my business problem most cost effectively? In many cases, we might ask whether an AI model is even necessary.
When AI is the right solution, user engagement from inception is critical. This helps to ensure that the users’ functional and emotional goals are met. In the end, it supports user acceptance of the AI and its recommendations.
Clearly internal users of business systems will have concerns about the impact of AI on their roles and jobs. It’s important not to take the users away from being actors in the process, and project them into the role of observers by focusing on the benefits that AI offers to them. Change management communications should be effective with users when the focus is on the organizational objectives for the business in using AI. Done correctly this moves towards engaging users in support of how that will happen.